- Docente: Fabio Tamburini
- Credits: 6
- SSD: L-LIN/01
- Language: Italian
- Teaching Mode: Traditional lectures
- Campus: Bologna
- Corso: Second cycle degree programme (LM) in Data, Methods and Theoretical Models For Linguistics (cod. 5946)
-
from Mar 31, 2025 to May 15, 2025
Learning outcomes
At the end of the course the student know in detail the main Deep Learning models used for natural language processing both in relation to written and spoken language. She/He also know the main technological frameworks useful for developing computational applications.
Course contents
- Machine/Deep Learning Recap
- Classification & Clustering using Scikit-Learn (SVC, KNN, Decision Trees, etc.)
- Technical details for training DNNs
- Activation functions
- Loss functions
- Optimisation & automatic gradient computation
- PyTorch framework
- LAB: How to use Colab for experiments
- LAB: Function optimisation in pytorch
- LAB: First DNN experiment using Multi-Layer Perceptrons
- LAB: Second DNN experiment using recurrent NN
- Transformers internal structure
- BERT & GPT
- Pre-Training and Fine-tuning a transformer
- LAB: BERT fine-tuning on GLUE benchmark
- Fine-tuning with Adapters (LoRA)
Students with SLD or temporary or permanent disabilities. It is suggested that they get in touch as soon as possible with the relevant University office (https://site.unibo.it/studenti-con-disabilita-e-dsa/en) and with the lecturer in order to seek together the most effective strategies for following the lessons and/or preparing for the examination.
Readings/Bibliography
Some chapters extracted from:
- Tamburini F. (2022). Neural Models for the Automatic Processing of Italian, Bologna: Pàtron.
- Raschka S., Liu Y., Mirjalili V. (2022). Machine Learning with Pytorch and Scikit-learn, Packt Publishing
- Stevens E., Antiga L., Viehmann T. (2021). Deep Learning With Pytorch, Manning.
Slides, handouts and papers downloadable from the course web site.
The course contents for students not attending the lessons are the same. However, students not able to attend the lessons are strongly invited to contact the teacher to get some explanations and avoid any misunderstanding about the course contents and reading materials.
Teaching methods
Face-to-face classes and laboratory sessions for 30 hours.
Assessment methods
The exam consists in the development of a project agreed with the teacher using the Google Colab environment, in the preparation of a written report that illustrates the project, in line with what was seen in class with respect to the experimental part, and in the relative discussion during the exam. The projects are designed to be carried out by 2 (TWO!) students. Any exceptions must be agreed with the teacher.
Reaching a clear view of all the course topics as well as using a correct language terminology will be valued with maximum rankings.
Mnemonic knowledge of the course topics or not completely appropriate terminology will be valued with intermediate rankings.
Unknown topics or inappropriate terminology use will be valued, depending on the seriousness of the omissions, with minimal or insufficient rankings.
It is compulsory to register for the exam using the online [https://almaesami.unibo.it/almaesami/welcome.htm] procedure.
Students with SLD or temporary or permanent disabilities. It is necessary to contact the relevant University office (https://site.unibo.it/studenti-con-disabilita-e-dsa/en) with ample time in advance: the office will propose some adjustments, which must in any case be submitted 15 days in advance to the lecturer, who will assess the appropriateness of these in relation to the teaching objectives.
Teaching tools
The course web site is the central point for any kind of information about the course. It contains the handouts and the readings discussed during the lessons as well as a rich software repository useful for laboratory practice.
Links to further information
https://corpora.ficlit.unibo.it/MNNLP/
Office hours
See the website of Fabio Tamburini
SDGs
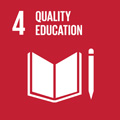
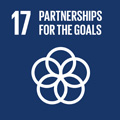
This teaching activity contributes to the achievement of the Sustainable Development Goals of the UN 2030 Agenda.